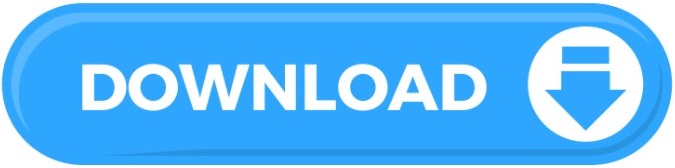

Basically, it is the process of determining where chunks of logic or memory should be placed on a chip in order to maximize the chip’s operating frequency while minimizing its power consumption and the area it takes up. The problem in question is called placement. “Our goal has been clarity of understanding that will allow the community to move on,” he told engineers. That’s especially notable given that the experiments did not allow Circuit Training to use its signature ability-to improve its performance by learning from other chip designs. In most cases, Circuit Training was not the winner, but it was competitive. Kahng declined to speak with IEEE Spectrum for this article, but he spoke to engineers last week at ISPD, which was held virtually. Using Google’s open-source version of its process, called Circuit Training, and reverse-engineering some parts that were not clear enough for Kahng’s team, they set reinforcement learning against a human designer, commercial software, and state-of-the-art academic algorithms. He and colleagues at the University of California, San Diego, delivered what he called “an open and transparent assessment” of Google’s reinforcement learning approach. This year at that same conference, a leader in the field, IEEE Fellow Andrew Kahng, hoped to put an end to the acrimony once and for all. It pitted established male electronic design automation (EDA) experts against two young female Google computer scientists, and the underlying argument had already led to the firing of one Google researcher. It was described by observers as a “trainwreck” and an “ambush.” The crux of the clash was whether Google’s AI solution to one of chip design’s thornier problems was really better than those of humans or state-of-the-art algorithms.

But a year ago at the International Symposium on Physical Design (ISPD), things got out of hand.
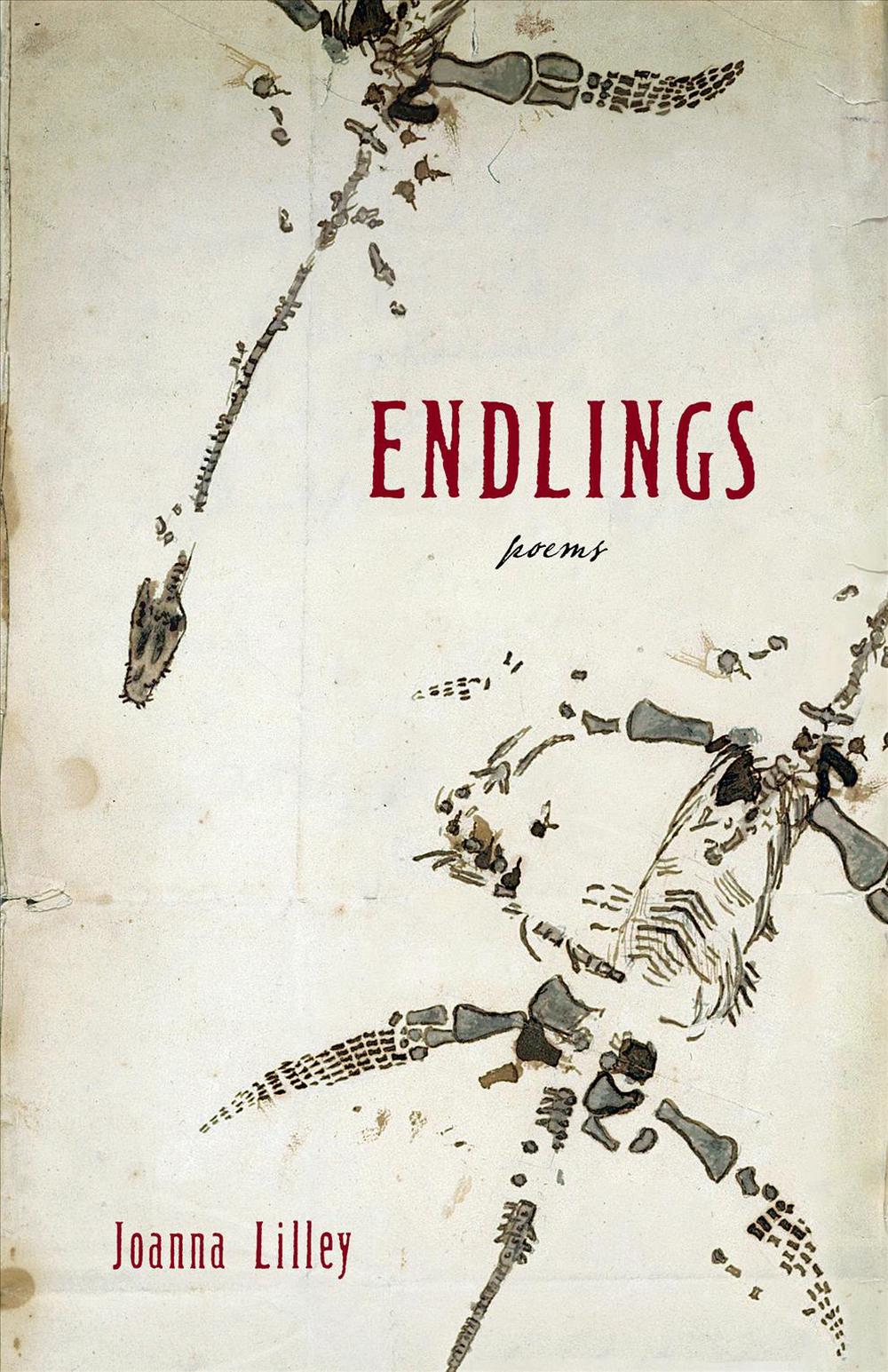
Discussions at chip design conferences rarely get heated.
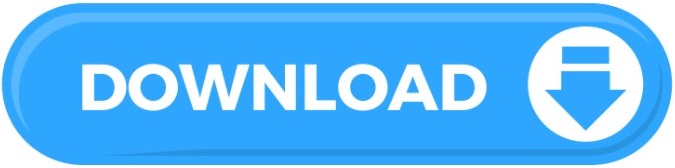